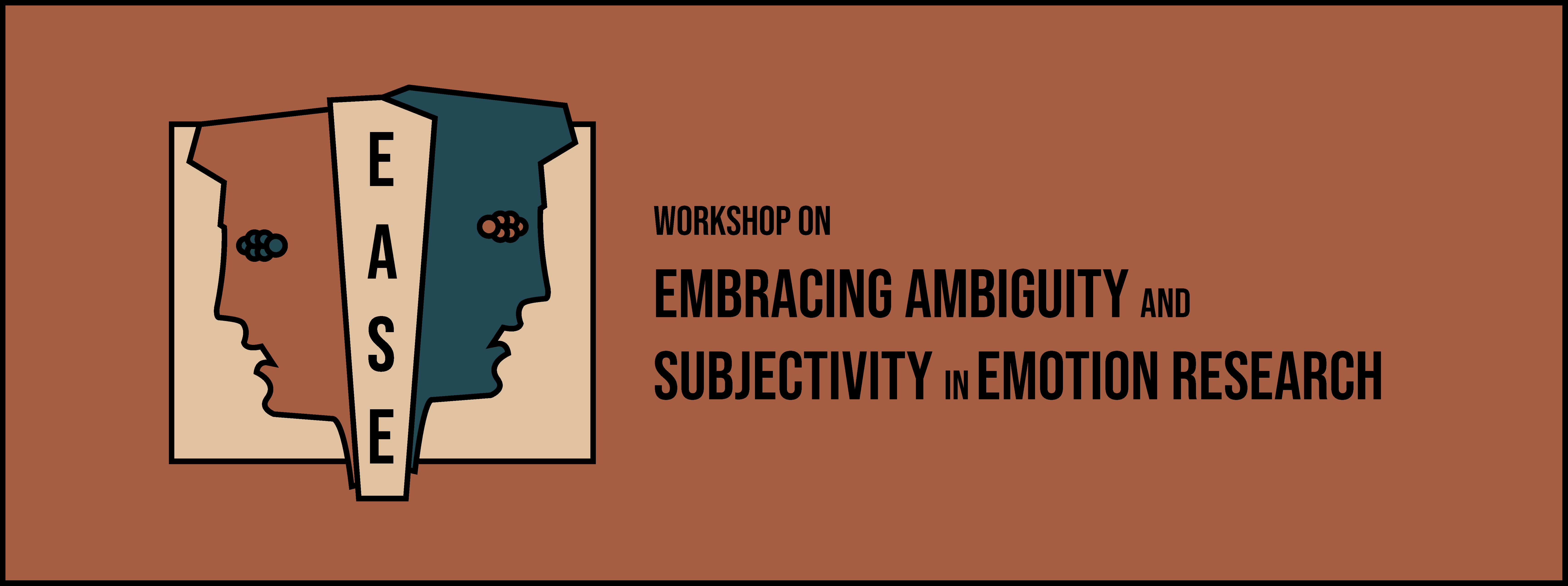
About
Emotion research and affective computing involve tasks like describing, predicting, and explaining emotional reactions to stimuli such as text, images, and audio. A prevalent assumption in this field is that emotional reactions can be adequately represented by a single numerical value or label. However, we contend that variability is common in this domain due to the “subjectivity” (individuals can have vastly different responses to the same stimulus) and “ambiguity” (the same response could be described in multiple, equally valid ways) inherent in emotional processes. These properties make assumptions of a singular true description for responses highly problematic for research and applications, such as emotion recognition, affective content analysis, or generating affective behavior in robots or virtual agents. Consequently, we are convinced that considering subjectivity and ambiguity is crucial and that doing so adequately will require a deeper understanding of these phenomena and the development of alternative practices (e.g., modeling responses as distributions over several viable outcomes, or using contextual variables for disambiguation).
This workshop aims to increase awareness of and appreciation for the importance of ambiguity and subjectivity in the affective computing community. In particular, we hope to facilitate focused discussions on relevant issues and support future research on these important concepts by providing a multi-disciplinary forum for interested researchers and practitioners.
Topics of interest for the workshop are position papers touching on one of the following KEY THEMES:
- Theoretical Foundations: Discussions and refinement of terminology related to ambiguity and subjectivity, as well as integrations of relevant perspectives from areas like psychology, statistics, and philosophy.
- Database and Annotation Design: Proposals, discussions, or comparisons of different approaches to data collection and annotation to facilitate the study of ambiguity and subjectivity, e.g., stimulus types, measurement instruments, annotation software, and emotion representations.
- Modeling and Evaluation Approaches: Proposals, discussions, or comparisons of different approaches to modeling and model evaluation that consider ambiguity or subjectivity.
- Future Challenges and Opportunities: Discussions of questions, directions, and applications for future research on ambiguity and subjectivity to explore.
Important Dates
All deadlines are at the end of the day in the GMT-12 timezone.
Submission Deadline: | |
Acceptance Notifications: | |
Camera-ready Deadline: | |
Workshop date: | 15 September, 2024 |
The EASE workshop is a satellite event of the 13th International Conference on Affective Computing and Intelligent Interaction (ACII 2024) on 15 September 2024. For details, see: https://acii-conf.net/2024/
Submission
We invite submissions of Position Papers (max. 5 pages; 4 pages + 1 page for references) focusing on one of the four key themes mentioned in the introduction, i.e., (1) Theoretical Foundations, (2) Database and Annotation Design, (3) Modeling and Evaluation Approaches, and (4) Future Challenges and Opportunities.
Submissions should be double-blind, i.e., anonymous, follow the official submission guidelines from ACII2024, and clearly state which one of the four key themes they most closely align with. Each paper will be sent to at least two expert reviewers associated with relevant key areas and will have one of the organizers assigned as editor.
Accepted submissions will be published in the workshop proceedings of ACII 2024. At least one author must register for the workshop and one conference day.
Papers can be submitted via ACII’s EasyChair platform (choose track “Workshop: Embracing Ambiguity and Subjectivity in Emotion Research (EASE)”).
Program
Time slots will correspond to the local time in Glasgow, UK.
Location: Room ARC-237C, The Mazumdar-Shaw Advanced Research Centre, 11 Chapel Lane, University of Glasgow, G11 6EW
THEORETICAL FOUNDATIONS | |
09:00 – 10:00 | Invited Talk and Q&A Jeffrey Girard |
10:00 – 10:30 | Paper Presentation and Q&A Indeterminacy in Affective Computing: Considering Meaning and Context in Data Collection Practices Bernd Dudzik, Tiffany Matej Hrkalovic, Chenxu Hao, Chirag Raman and Masha Tsfasman. |
10:30 – 11:00 | COFFEE BREAK |
MODELING AND EVALUATION APPROACHES | |
11:00 – 12:00 | Invited Talk and Q&A Phil Woodland |
12:00 – 12:30 | Paper Presentation and Q&A Emotion Recognition Systems Must Embrace Ambiguity Jingyao Wu, Ting Dang, Vidhyasaharan Sethu and Eliathamby Ambikairajah |
12:30 – 13:30 | LUNCH BREAK |
DATABASE AND ANNOTATION DESIGN | |
13:30 – 14:30 | Invited Talk and Q&A Georgios N. Yannakakis — Embracing Human Feedback via Games |
14:30 – 15:00 | Paper Presentation and Q&A Embracing Subjectivity in Affective Research: Naturalistic and Controlled Settings Dominika Kunc, Joanna Komoszyńska, Stanisław Saganowski, Przemysław Kazienko, Karen S. Quigley and Lisa Feldman Barrett |
15:00 – 15:30 | Paper Presentation and Q&A Recommendations for Managing Ambiguities in Emotion Annotations Anargh Viswanath, Jelena Mallick, Teena Hassan and Hendrik Buschmeier |
15:30 – 16:00 | COFFEE BREAK |
FUTURE CHALLENGES AND OPPORTUNITIES | |
16:00 – 16:30 | Plenary Discussion |
16:30 – 17:00 | Closing and Future Planning |
Invited Speakers
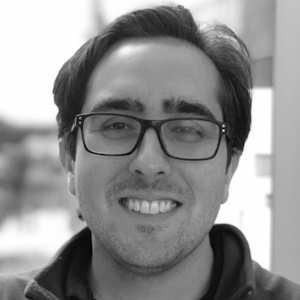
Jeffrey M. Girard
University of Kansas
Talk title: TBA
Talk abstract: TBA
Bio: Dr. Girard studies how emotions are expressed through verbal and nonverbal behavior, as well as how interpersonal communication is influenced by individual differences (e.g., personality and mental health) and social factors (e.g., culture and context). This work is deeply interdisciplinary and draws insights and tools from various areas of social science, computer science, statistics, and medicine.
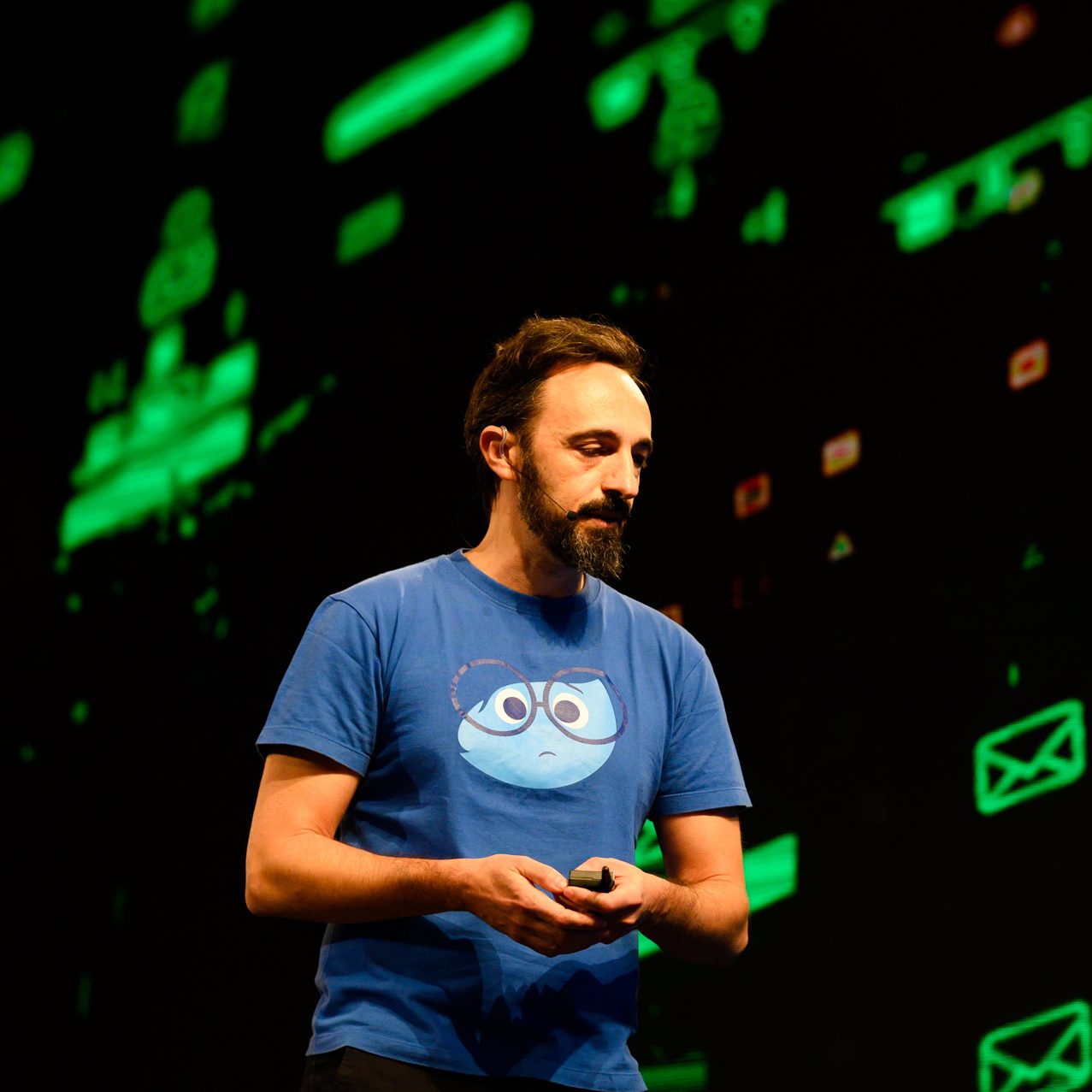
Georgios N. Yannakakis
University of Malta
Talk title: Embracing Human Feedback via Games
Talk abstract: Why is subjective and ambiguous human feedback such a critical element of every aspect of AI nowadays? What are the opportunities video games offer for the reliable collection of subjective human feedback and eventually the modeling of ambiguous phenomena such as affect? How can human feedback help us test games, represent the games we play, design creative AI algorithms, offer agency to AI, and ultimately understand player experience? In this talk, I will attempt to address these questions through a series of research studies that have led to a number of methods, tools and protocols for the reliable annotation and modeling of human feedback, in games and beyond.
Bio: Georgios N. Yannakakis is a Professor at the Institute of Digital Games, University of Malta, and a co-founder and research director of modl.ai (Malta). He is a leading expert of the game artificial intelligence research field with core contributions in machine learning, evolutionary computation, affective computing and player modelling, computational creativity and procedural content generation. He has published over 350 journal and conference papers in the aforementioned fields and his work has received several awards from top-tier academic conferences and journals. His research has been supported by numerous European grants and has appeared in Science Magazine and the New Scientist among other venues. Georgios has been involved in a number of journal editorial boards and he is currently the Editor in Chief of the IEEE Transactions on Games and an Associate Editor of the IEEE Transactions on Evolutionary Computation. He is the co-author of the Artificial Intelligence and Games textbook and the co-organiser of the Artificial Intelligence and Games summer school series. Georgios is an IEEE Fellow.
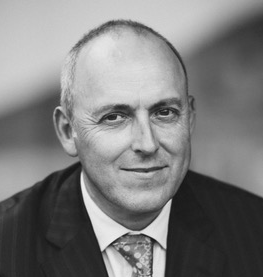
Phil Woodland
University of Cambridge
Talk title: Modelling Annotation Ambiguity in Spoken Emotion Data
Talk abstract: Different speakers and contexts (linguistic, social, dialogue) can cause large differences in emotion expression. Furthermore, different listeners perceive certain emotions again based on differing contexts, and hence, there is inherent variability in the annotation labels of spoken emotion data. Often, data that doesn’t have a majority-agreed label is ignored in class-based spoken emotion recognition, even though this data can be valuable. This talk first describes some techniques to model and evaluate the handling of nonmajority agreed data via extra classes, the use of evidential deep learning (EDL) to quantify uncertainty, and also extending EDL to model emotion distributions. An EDL-based approach is also developed and applied for attribute-based emotion annotations. An alternative approach of variability aware human annotator simulation is then discussed based on conditional softmax flow (for classes) and conditional integer flow (for attributes). It is demonstrated that the approaches discussed can both yield excellent classification accuracy/mean prediction as well as matching the distribution of human annotators. The talk concludes with conclusions and outlook.
Bio: Prof. Woodland’s research is in the area on speech and language technology with a major focus on developing all aspects of speech recognition systems. After working with British Telecom Research Labs for three years, he returned to a Lectureship at the University of Cambridge in 1989 and became a Reader in 1999 and a (full) Professor in 2002. He has authored or couthored more than 250 papers in the area of speech and language technology with a main focus on speech recognition systems. He was the recipient of number of Best Paper awards including for work on speaker adaptation and discriminative training. He is one of the original coauthors of the HTK toolkit and has continued to play a major role in its development. He was a Member of the Editorial Board of Computer Speech and Language (1994–2009) and is currently a Member of the Editorial Board Member of Speech Communication. He is a Fellow of the International Speech Communication Association, the IEEE and the Royal Academy of Engineering. His group has developed a number of techniques that have been widely used in large vocabulary systems including standard methods for transform-based adaptation and discriminative sequence training. He has worked on the use of deep neural networks for both acoustic models and language models. His current work has a focus on the use and development of end-to-end trainable neural network systems.
Organizing Committee
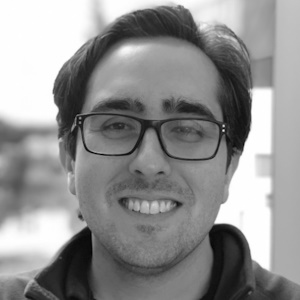
Jeffrey M. Girard
jmgirard@ku.edu
(University of Kansas)
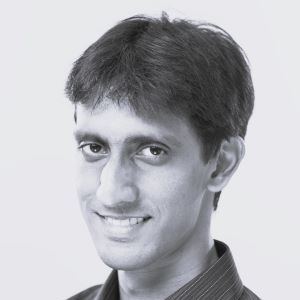
Vidhyasaharan Sethu
v.sethu@unsw.edu.au
(University of New South Wales)
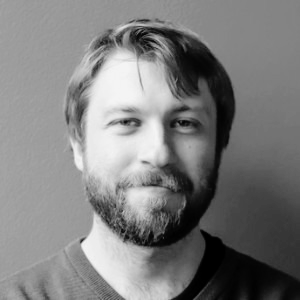
Bernd Dudzik
b.j.w.dudzik@tudelft.nl
(Delft University of Technology)
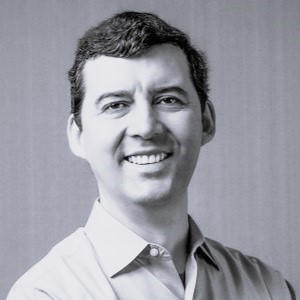
Carlos Busso
busso@utdallas.edu
(University of Texas at Dallas)
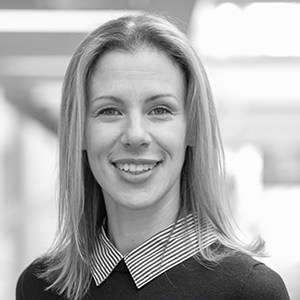
Emily Mower Provost
emilykmp@umich.edu
(University of Michigan)
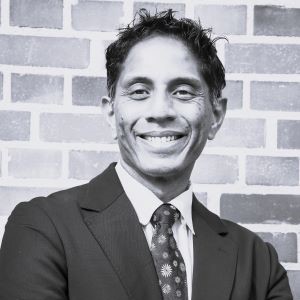
Shrikanth Narayanan
shri@usc.edu
(University of Southern California)
Program Committee
- Agata Lapedriza (Universitat Oberta de Catalunya)
- Chi-Chun Lee (National Tsing Hua University)
- Chenxu Hao (Delft University of Technology)
- Dennis Küster (Bremen University)
- Einat Liebenthal (Harvard Medical School)
- Ting Dang (University of Melbourne)